Image-based target identification on a tiny Risc-V multi-core application processor
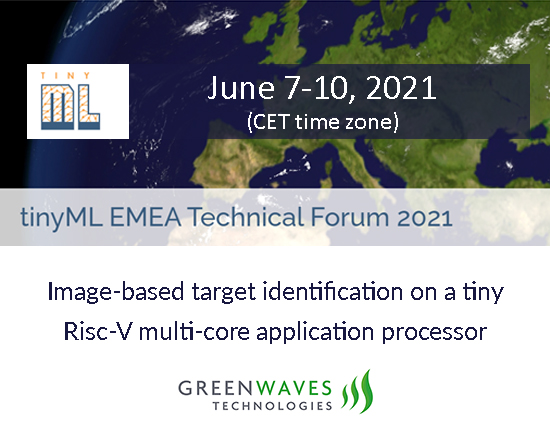
Next-generation battery-operated smart cameras will feature a miniaturized form factor and AI capabilities to infer high-level information from the surrounding environment. Because of the tight power envelope and the high computational cost of top-accurate image-based recognition models, the processing engine must reach an extremely high level of energy efficiency. Lastly, high flexibility, which is gained by means of software programmability, becomes essential to support a wide range of applications and algorithms, and, in particular, in the Deep Learning space.
In this presentation, we present our methodology to embed sophisticated Deep Learning based image processing pipelines. Our design flow targets the GAP application processor, a TinyML processing platform that includes 8 general purpose RISC-V cores featuring a DSP-oriented ISA. To enable complex end-to-end image-based pipelines for target identification on such a tinyML processor, we have developed an SW toolset that generates optimized parallel C code based on complex DNN topologies. We experimentally test our methodology on multiple smart-camera use cases ranging from head pose detection, vehicle and people spotting or license plate recognition, to demonstrates the unprecedented level of energy efficiency for top-accurate models if compared to traditional single-core MCUs.